TCDModelSamplingDiscrete: An In-Depth Guide
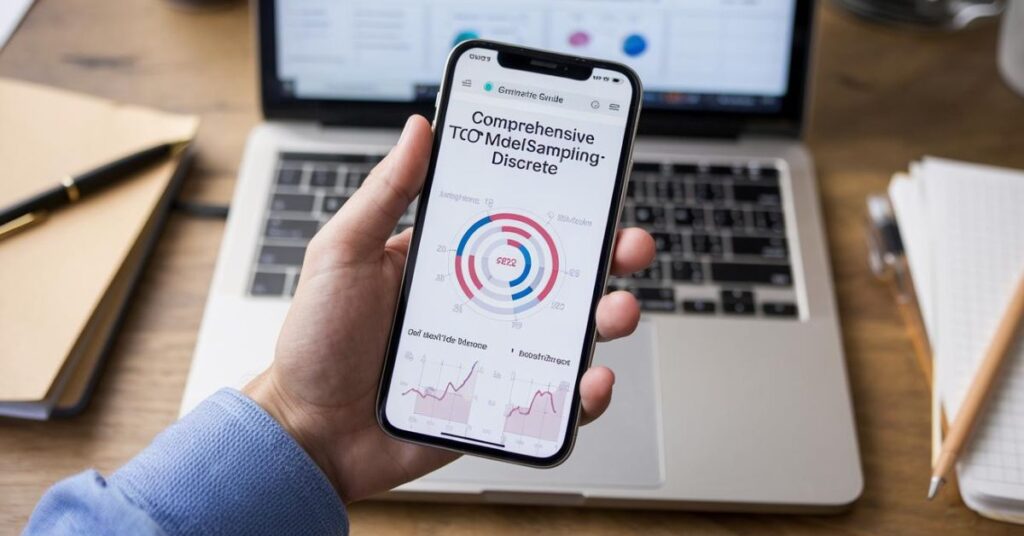
In the evolving landscape of data analysis and modeling, TCDModelSamplingDiscrete stands out as a revolutionary approach to discrete sampling. This model simplifies and enhances the way discrete systems are sampled and modeled, making it an invaluable tool for researchers, analysts, and professionals across various fields. This comprehensive guide explores TCDModelSamplingDiscrete in detail, examining its key features, benefits, applications, and future potential. We’ll also address common questions to provide a thorough understanding of this technology.
What is TCDModelSamplingDiscrete?
TCDModelSamplingDiscrete is an advanced framework designed to improve the process of sampling discrete data. Discrete sampling involves selecting distinct data points from a larger set for analysis or modeling, as opposed to continuous sampling, which deals with data that changes smoothly over a range. This model offers a refined method for handling discrete data, aiming to provide higher accuracy and efficiency compared to traditional sampling techniques.
Key Features of TCDModelSamplingDiscrete
- Enhanced AccuracyOne of the primary advantages of TCDModelSamplingDiscrete is its precision. The model utilizes sophisticated algorithms and methods to minimize sampling errors, ensuring that the sampled data is as close to the true values as possible. This high level of accuracy is crucial for making reliable predictions and informed decisions based on discrete data.
- Simplified Sampling ProcessTraditional discrete sampling methods can be complex and require specialized knowledge. TCDModelSamplingDiscrete addresses this issue by simplifying the sampling process. It reduces the need for extensive manual intervention and complex computations, making it more accessible to users with varying levels of expertise.
- Increased EfficiencyEfficiency is another significant benefit of TCDModelSamplingDiscrete. The model optimizes the sampling process to reduce computational time and resource usage. This is particularly advantageous when dealing with large data sets or when rapid sampling is required for time-sensitive applications.
- Flexibility and VersatilityTCDModelSamplingDiscrete is designed to be adaptable to different types of discrete data and systems. Its versatility allows it to be applied across a range of fields, from scientific research to industrial quality control. This flexibility makes it a valuable tool for many different applications.
Applications of TCDModelSamplingDiscrete
1. Academic and Industrial Research
In research settings, whether academic or industrial, accurate data sampling is crucial. TCDModelSamplingDiscrete facilitates the analysis of discrete data sets, enabling researchers to obtain reliable samples and draw valid conclusions. This model is particularly useful in fields like statistics, engineering, and social sciences.
2. Quality Control in Manufacturing
For manufacturers, discrete sampling is an essential part of quality control. TCDModelSamplingDiscrete helps in sampling product batches to ensure they meet quality standards. By providing accurate and efficient sampling, it helps maintain high product quality and reduce defects.
3. Financial Modeling and Risk Assessment
In the financial sector, discrete sampling is used to evaluate various financial instruments and risks. TCDModelSamplingDiscrete enhances the precision of financial models, which is vital for assessing investment opportunities, managing risks, and making strategic financial decisions.
4. Computer Science and Algorithms
Computer scientists and engineers use discrete sampling to test and optimize algorithms and data structures. TCDModelSamplingDiscrete provides an efficient way to sample data for computational tasks, improving algorithm performance and reliability.
Advantages of TCDModelSamplingDiscrete Over Other Solutions
1. Superior Precision
Compared to conventional discrete sampling methods, TCDModelSamplingDiscrete offers superior precision. Its advanced algorithms reduce sampling errors and provide more accurate results, which is crucial for applications that rely on precise data.
2. Simplified Implementation
Traditional discrete sampling techniques often involve complex procedures and require specialized knowledge. TCDModelSamplingDiscrete simplifies these processes, making it easier for users to implement and use effectively without needing extensive training.
3. Faster Processing
The efficiency of TCDModelSamplingDiscrete translates to faster processing times. This is especially beneficial in scenarios where large volumes of data need to be sampled quickly, such as in real-time systems or large-scale research projects.
4. Broad Applicability
Unlike some discrete sampling models that are limited to specific applications, TCDModelSamplingDiscrete is highly versatile. It can be applied across various industries and research areas, making it a valuable tool for a wide range of users.
Challenges and Considerations
1. Learning Curve
While TCDModelSamplingDiscrete offers many benefits, there is a learning curve associated with its use. New users may need time to familiarize themselves with the model’s features and how to apply it effectively in their specific contexts.
2. Data Quality
The effectiveness of TCDModelSamplingDiscrete depends on the quality of the input data. Poor-quality data can lead to inaccurate sampling results. Therefore, it is essential to ensure that the data being sampled is accurate, complete, and representative.
3. Implementation Costs
Adopting TCDModelSamplingDiscrete may involve costs related to software, training, and integration into existing systems. Organizations need to consider these costs when planning to implement this model.
Future Prospects
The future of TCDModelSamplingDiscrete is promising, with potential advancements on the horizon. As technology continues to evolve, we can expect further improvements in accuracy, efficiency, and user-friendliness. Future developments may also expand its applications, making it an even more valuable tool for a broader range of industries and research fields.
1. Technological Advancements
Advances in computing power and algorithms may lead to even more precise and efficient versions of TCDModelSamplingDiscrete. These improvements could enhance its performance and broaden its applicability.
2. Expanded Applications
As new fields and industries emerge, TCDModelSamplingDiscrete could be adapted to address new challenges and opportunities. Its versatility positions it well for continued relevance and usefulness in various domains.
3. Enhanced User Experience
Future updates may focus on improving the user experience by making the model more intuitive and easier to use. This could involve developing better user interfaces, providing more comprehensive training resources, and integrating with other tools and systems.
FAQs
Q1: What are the main benefits of TCDModelSamplingDiscrete?
A1: The main benefits of TCDModelSamplingDiscrete include enhanced accuracy, simplified sampling processes, increased efficiency, and flexibility. It provides precise sampling with reduced complexity and faster processing times.
Q2: How does TCDModelSamplingDiscrete improve upon traditional discrete sampling methods?
A2: TCDModelSamplingDiscrete improves upon traditional methods by offering superior precision, simplified implementation, and faster processing. Its advanced algorithms and efficient processes set it apart from older techniques.
Q3: In what industries can TCDModelSamplingDiscrete be used?
A3: TCDModelSamplingDiscrete can be used in various industries, including academic and industrial research, manufacturing quality control, financial modeling, and computer science. Its versatility makes it suitable for a wide range of applications.
Q4: What challenges might users face when adopting TCDModelSamplingDiscrete?
A4: Users may face challenges such as a learning curve, data quality issues, and implementation costs. Ensuring high-quality data and investing in proper training and integration are essential for effective use of the model.
Q5: What does the future hold for TCDModelSamplingDiscrete?
A5: The future of TCDModelSamplingDiscrete looks promising, with potential advancements in technology and applications. Future developments may lead to more precise, efficient, and user-friendly versions of the model, expanding its usefulness across various fields.
Conclusion
TCDModelSamplingDiscrete represents a significant advancement in the field of discrete sampling. Its enhanced accuracy, simplified processes, and efficiency make it an invaluable tool for a wide range of applications. By addressing common challenges and leveraging its advantages, users can greatly improve their discrete sampling and modeling efforts. As technology continues to evolve, TCDModelSamplingDiscrete is poised to play an increasingly important role in various industries and research fields, making it a key component of modern data analysis and decision-making.